In the previous blog we discussed some of the metrics that we felt needed to rbe explored in order to carry out a fuller evaluation of ASR recordings in order to try to address some of the issues occurring in the output to captions and transcriptions.
Recently we have developed a range of practical metrics evaluated by a series of scores and value-added comments. This was felt necessary to solve the issue of selection bias in ASR that seems to highlight errors due to pronunciation differences affected by age, gender, disability, accents and English as a foreign language when listening to lecturers across a range of subjects. It is hoped these can be addressed by providers using differently biased input data that is customized, instead of using one single accuracy percentage to denote the performance of the ASR services. Evaluators also need to be aware of these issues and suggest the need for more inclusive training data to enable corrections to automatically occur in a proactive manner.
In the table below the list of items that may be used in a review has been expanded well beyond those usually used to find the type of word errors, omissions or additions that are occurring.
Speaker Speech | Environment Noise | Content – What is expressed | Technology Hardware | Recording |
Pronunciation Clarity Speed Loudness Pitch Intonation Inflection Accent, Age, Gender, Use of Technology Too far away / near the microphone | Ambient noise/continuous Reverberation Sudden noise Online/Offline User device Room system Conversation vs Presentation Single speaker Overlapping speakers Multi-speakers | Complexity Unusual names, locations, and other proper nouns Technical or industry-specific terms Out of Vocabulary / not in the dictionary Homonyms | Smart phone Tablet Laptop Desktop Microphone Array Headset Built-in Hand held Camera Specialist /Smart Computer Mobile | Direct audio recording Synthetic speech recording Noise-network distorted speech Connectivity Live / Real-Time Recorded |
When it comes to pronunciation or typical morphosyntactic differences in the way a language is used, developers may be able to pre-empt and automate corrections for consistent errors. An example includes articulation errors that are typical for those speaking English as a foreign language such as the omission of “th”, “v” and “rl” sounds that do not appear in some Chinese dialects.
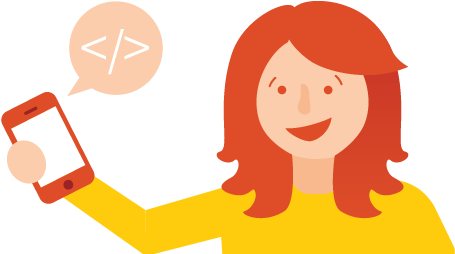
Age and gender biases could also be improved using semi-automated annotation systems, but speaker style remains an issue that is hard to change when there is direct ‘human-machine interaction’ rather than someone reading text.
Moreover, there still remains the manual process of checking for metrics, such as those that examine the way technology is used. This type of problem can be judged visually if the camera catches the interactions and in an auditory manner, such as walking away from the microphone or turning ones back to the camera etc. AI Video content analysis is moving apace and these techniques could help us in time!
Ultimately the training data is the main issue but automated bias mitigation techniques are being explored by researchers and the outcomes look promising and there also needs to be some swift designing of a more sophisticated and adaptable ASR performance metric evaluator to automate the process of reviewing output!